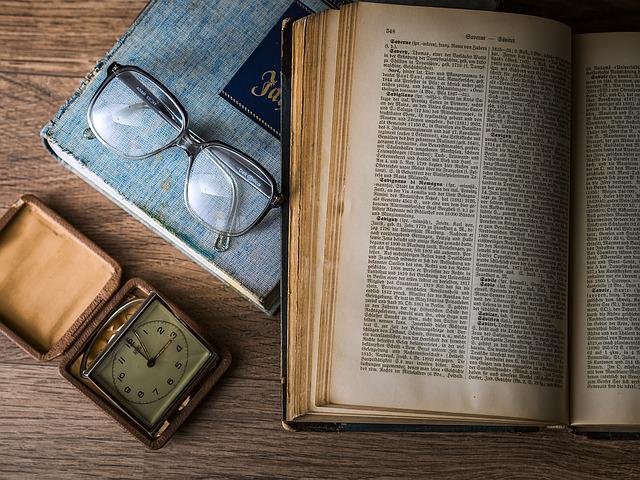
5 Facts About Sample Sizes in Research
A good sampling strategy requires a sufficiently large sample. A small sample size will inevitably yield poor statistical power. In addition, it can add to costs and make it difficult to estimate the standard deviation. To avoid these problems, many investigators choose to increase the sample size by at least 10 percent. This practice compensates for possible reasons for dropping out of a study, such as incomplete records, failure to obtain biological specimens, or any other study-related problem.
Table of Content
The sample size is a significant determinant of statistical power.
There are several ways to improve the statistical power of a research study. Statistical power is a critical issue, especially for studies based on theory. For example, alterations to the design can reduce unexplained errors. Analysis of covariance and matching designs increase sample homogeneity. A study’s design also has a significant impact on statistical power. Using alternative arrangements can increase power. Overall and Dalal (1965) discuss using prior estimates of mean squares and other methods to reduce unexplained error.
Researchers should estimate the statistical power of a study before conducting the actual data collection. Any report that contains results should state the power of the study to detect an effect of a given size. This allows readers to judge the efficacy of the research. Increasing sample size might cost more, but it may give researchers more reliable effect size estimates. However, larger sample sizes may not be necessary. You can also look for a new sample size example in research.
It isn’t easy to estimate the standard deviation.
As a researcher, you may wonder how to calculate the standard deviation for your sample size. You may find it difficult to estimate the standard deviation, as it’s one-fourth of the population’s standard deviation. There are some ways to calculate the standard deviation, however. The first approach involves taking half the population’s standard deviation and cutting it by four times. The second method estimates the standard deviation from published research in the scientific literature and from market research.
It is hard to estimate the standard deviation for sample sizes in research, but you can use a formula to calculate the standard deviation from historical data. However, historical data are often unavailable; thus, it is necessary to estimate the sample size by using average standard deviations across several studies. While smaller sample sizes are acceptable, it is best to avoid them when possible. However, when calculating sample size, remember that smaller samples tend to be less significant than large ones.
It can add to costs.
While larger sample sizes produce smaller margins of error, they also add to the research costs. While larger samples better represent the population, using too small a sample size can be expensive and time-consuming. A more accurate method for estimating sample size is to calculate total costs divided by the square root of the sample size. The fair root method ensures 90% power while being more economical than other sample sizes.
The conventional sample size approach specifies a goal, calculates the number of subjects needed to achieve it, and does not explicitly account for costs. Health-related research reviewers, however, often expect the use of 80% or 90% power in a study to meet ethical standards. Despite these expectations, many studies do not receive funding because of limited resources. A good peer-review process allows many studies to go unfunded.
It can be split into sub-samples.
A sampling unit represents a distinct element of a population. This can be an individual, a family, a household, or even a city or country. It is important to note that the sampling unit is not necessarily the same as the population under study. For instance, a study examining attitudes towards international students may want to sample international students from different regions of the country. However, a study with a larger sample size is more likely to yield more meaningful results.
Depending on the study, sample sizes can be as small as 1% of the total population. For descriptive research, a sample size of 20 percent of the population is appropriate. For experiments with multiple variables, a minimum of 30 samples is necessary. Sub-samples within the same study group must contain at least thirty subjects. If more than three variables are being studied, sample sizes must be more significant.
It can be an optimization process.
When selecting sample sizes for research studies, consider the study’s objectives. The primary purpose is to detect a clinically relevant effect, and most quantitative studies require a pre-study calculation. In addition, the number of patients included in a study is usually limited by time, cost, and ethical considerations. However, choosing the right size is essential, as a too-small sample may fail to detect an existing binding effect, while a large piece might waste time and resources. In some cases, optimizing the sample size to meet ethical approval may even be necessary.